Healthcare
Our AI software detects: annotation errors in data, outliers (anomalous patients/records), ambiguous cases, and bad decisions (mis-diagnoses/prescriptions).
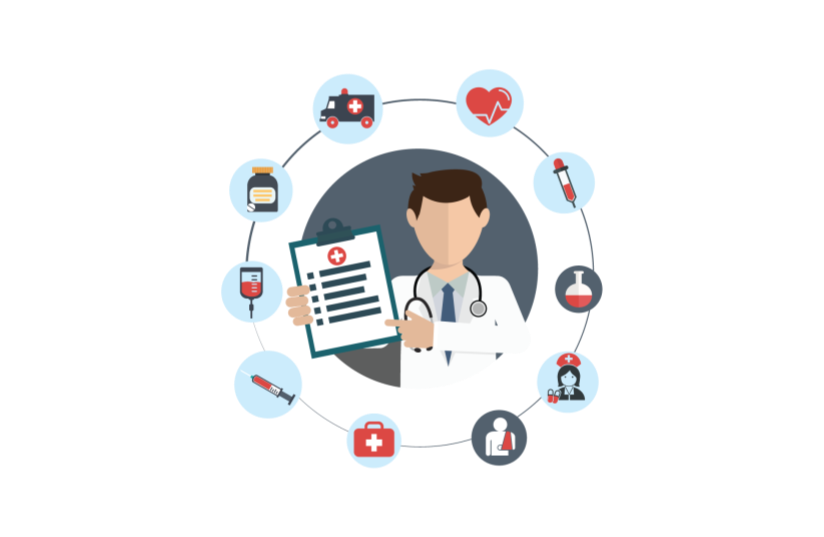
Case StudyShands Hospital at the University of Florida
We have developed pervasive sensing and data processing system which collects data from multiple modalities depth images, color RGB images, accelerometry, electromyography, sound pressure, and light levels in ICU for developing intelligent monitoring systems for continuous and granular acuity, delirium risk, pain, and mobility assessment.
Our approach is based on the Cleanlab implementation of active learning for data annotation
Our datasets include over 18 million depth image frames and 22 million patient face image frames extracted from videos. It is not practical to annotate the entirety of these massive datasets. Active learning is an important machine learning technique that involves an iterative process to choose most informative data samples to be labeled.
Another important aspect [of active learning] is the annotator quality, which can significantly impact the training effectiveness of the machine learning model.
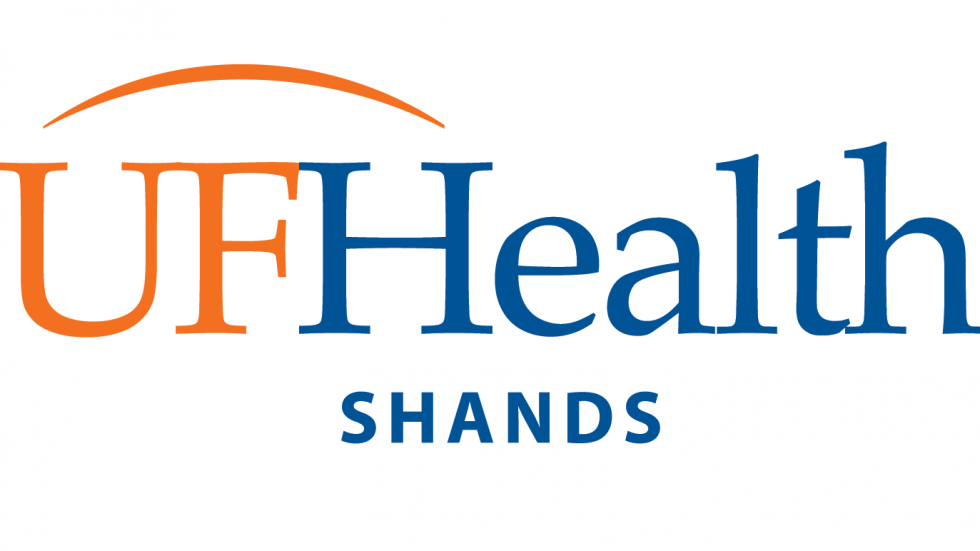
HOW CLEANLAB HELPS WITH HEALTHCARE DATA HYGIENE
Videos on using Cleanlab Studio to find and fix incorrect labels for:
- text data (e.g. medical reports)
- image data (e.g. medical imaging)
Automatically discover outliers (anomalies) which may have an outsized impact on data-driven conclusions and should be handled with care. Read more.
Model images together with tabular (numeric, categorical) and text information. Cleanlab’s AI helps you correct errors in electronic health records, insurance documents, patient communications, and medical imaging metadata/annotations.
Know which subset of the data is high-quality with confidence, and evaluate the quality of different data sources.
Effectively analyze data labeled by multiple annotators (clinicians), and estimate which examples require additional review and which annotators are best/worst overall. Read more.
Use our ActiveLab system (active learning with relabeling) to efficiently collect new data labels for training accurate models.
Automatically train robust machine learning models using complex healthcare datasets. Deploy them with 1-click to make predictions and catch bad decisions in real-time. Learn about the Data-Centric AI techniques used to produce robust models via the free MIT course taught by the Cleanlab founders: https://dcai.csail.mit.edu/
Auto-correct common issues in messy healthcare data to immediately produce more reliable ML models:
Related applications
Foundation and Large Language Models
Boost fine-tuning accuracy and reduce time spent
Data Annotation & Crowdsourcing
Find the optimal consensus label and quality of each annotator.
Data Entry, Management, and Curation
AI expert review of your data stores to find errors or incorrect labels.
Business Intelligence / Analytics
Correct data errors for more accurate analytics/modeling enabling better decisions.
Customer Service
Produce better data/models faster for customer support, customer segmentation, ...