Financial Services and Insurance
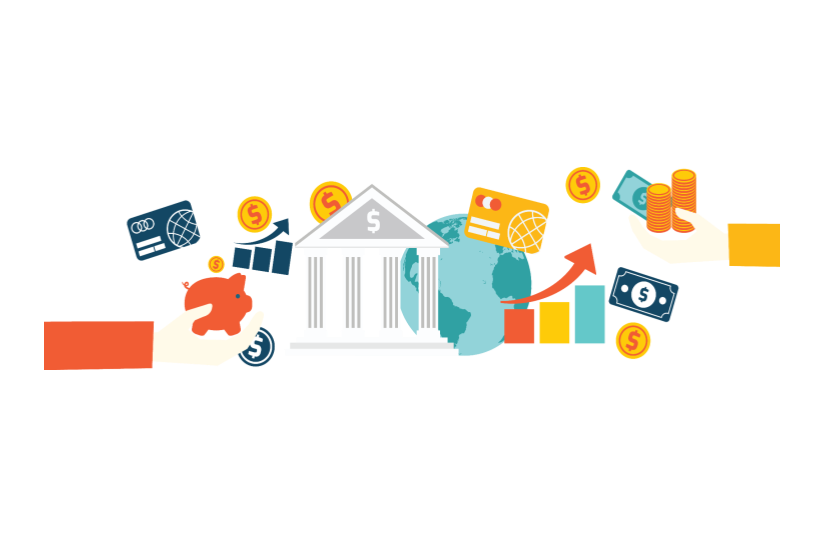
Case StudyBanco Bilbao Vizcaya Argentaria (BBVA)
[We used Cleanlab in] an update of one of the functionalities offered by the BBVA app: the categorization of financial transactions. These categories allow users to group their transactions to better control their income and expenses, and to understand the overall evolution of their finances. This service is available to all users in Spain, Mexico, Peru, Colombia, and Argentina.
We used AL [Active Learning] in combination with Cleanlab.
This was necessary because, although we had defined and unified annotation criteria for transactions, some could be linked to several subcategories depending on the annotator’s interpretation. To reduce the impact of having different subcategories for similar transactions, we used Cleanlab for discrepancy detection.
With the current model, we were able to improve accuracy by 28%, while reducing the number of labeled transactions required to train the model by more than 98%.
CleanLab assimilates input from annotators and corrects any discrepancies between similar samples.
CleanLab helped us reduce the uncertainty of noise in the tags. This process enabled us to train the model, update the training set, and optimize its performance. The goal was to reduce the number of labeled transactions and make the model more efficient, requiring less time and dedication. This allows data scientists to focus on tasks that generate greater value for customers and organizations.
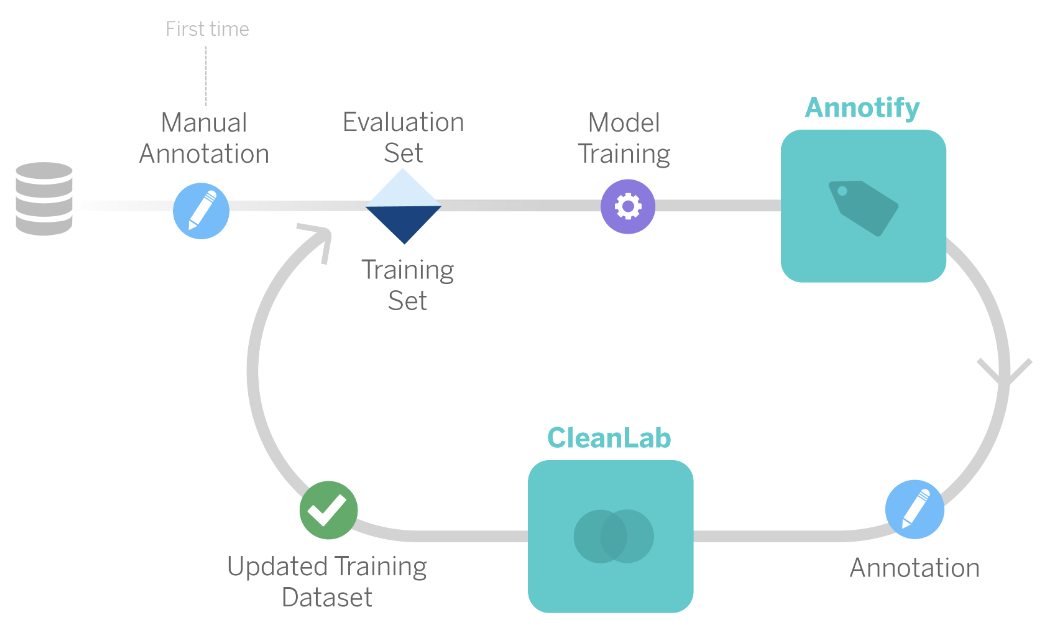
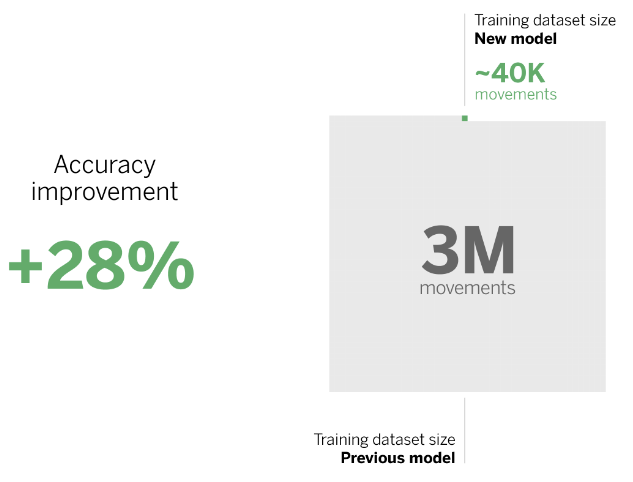
Case StudyWells Fargo
We used cleanlab for finding label errors in financial text data, helping us find label errors in our human annotation process. I like cleanlab more than alternative solutions because it's like 'bring your own model' & 'bring your own data', by acting like a wrapper around your model, it's superbly easy to implement, and it works well even when the model itself is not decent in classification due to relatively high noise rate (40% noisy) to achieve a consistent f1 score around 80%.
Case StudyCustomer Requests at Online Bank
· To automatically triage future customer requests, intent classification is a standard Machine Learning task in customer service applications that requires well-labeled data. When run on a dataset of customer requests (text) annotated with 10 different intents, Cleanlab Studio automatically revealed that over 5% of the dataset labels were incorrect. Cleanlab also automatically identified several out-of-scope requests, queries that cannot really be categorized as any of the intents in the dataset, including the following requests: "how much is 1 share of aapl", "is android better than iphone".
· This dataset was also used for customer analytics, to determine the relative frequencies of different types of customer requests and which types are most common. However some conclusions drawn from the original dataset areinaccurate due to the mislabeling and out-of-scope issues. Significantly more accurate conclusions were obtained by running the same analytics on the cleaned version of the dataset obtained from Cleanlab Studio.
· Businesses striving to make better decisions for customers must rely on accurate data-driven conclusions. These in turn rely on accurate data, which for this customer service application was easy to ensure with Cleanlab Studio.
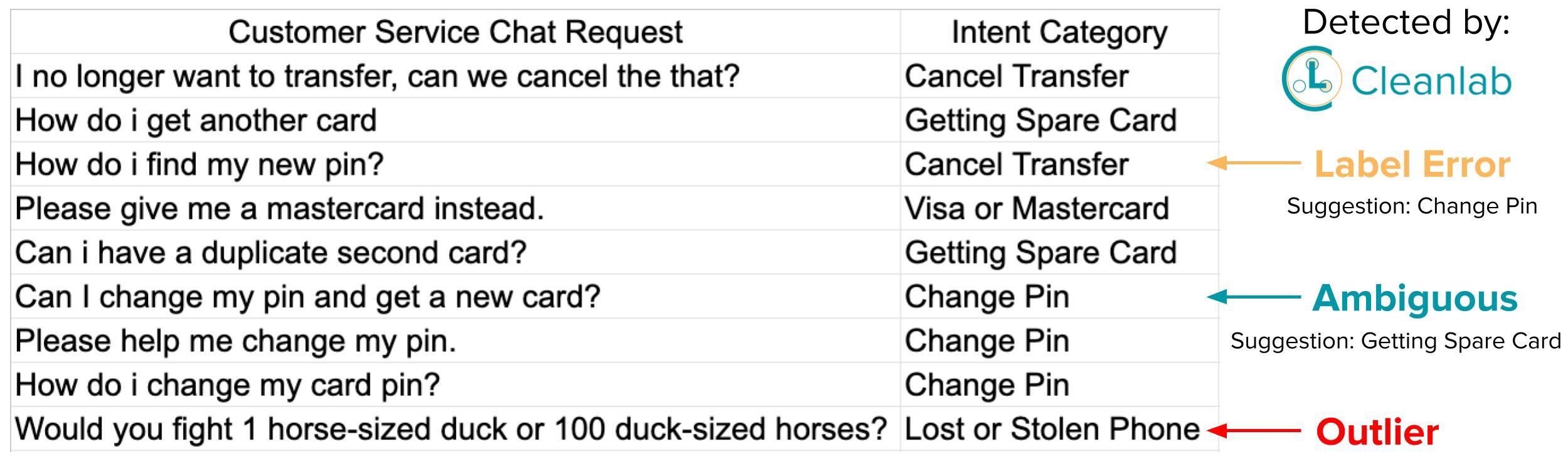
HOW CLEANLAB IMPROVES KEY FINANCIAL PROCESSES
Fraud Detection
Real-world training datasets often contain significant label noise, including overlooked cases of fraud. Cleanlab algorithms automatically detect and correct instances that are mislabeled, improving the reliability of fraud detection model training and evaluation.
Risk Assessment
Cleanlab Studio enables data-centric AI to build accurate ML models for messy real-world tabular or text data. You can effortlessly harness AutoML for various data types, including text, image, and tabular formats (Excel, CSV, Json), allowing you to focus on the most important aspect: the data. Learn more:
Outlier & Anomaly Detection
Utilize advanced AI for outlier detection in text, image, and tabular data (Excel, CSV, Json). Improve modeling of transactions, money reserves, and customers/providers. Move beyond basic statistics and historical fluctuations — Cleanlab Studio considers all available information for more effective anomaly diagnosis. Learn more about outlier detection with Cleanlab:
Aggregating Decisions/Predictions from Multiple Analysts
Cleanlab can analyze data annotated with multiple individuals’ decisions and estimate:
- A consensus decision for each instance that aggregates the individual decisions.
- A quality score for each consensus decision to gauge confidence that it is the correct choice.
- A quality score for each annotator to quantify their overall skills.
Video on using Cleanlab Studio to find and fix incorrect labels and anomalies in text data
Related applications
Data Entry, Management, and Curation
AI expert review of your data stores to find errors or incorrect labels.
Business Intelligence / Analytics
Correct data errors for more accurate analytics/modeling enabling better decisions.
Customer Service
Reduce cost/time to improve customer service entity recognition and ...
Foundation and Large Language Models
Boost fine-tuning accuracy and reduce time spent
Data Annotation & Crowdsourcing
Label data efficiently and accurately, understand annotator quality.