Automatically find and fix errors in your real-world data.
Turn unreliable data into reliable models and insights.
No Code.
Automated.
Data curation for LLMs
and the Modern AI stack.
Pioneered at MIT and trusted by hundreds
of top organizations, including:
Solutions
Cleanlab Studio handles the entire data quality and data-centric AI pipeline in a single framework for analytics and machine learning tasks.
Automatically improve your dataset. No code required.
Find and fix label issues (e.g incorrectly labeled data)
Find and fix data issues (e.g. outliers, ambiguous data)
Know when to trust your data: Avoid spending time/cost to review data that’s already high quality, by automatically validating all datasets in Cleanlab Studio
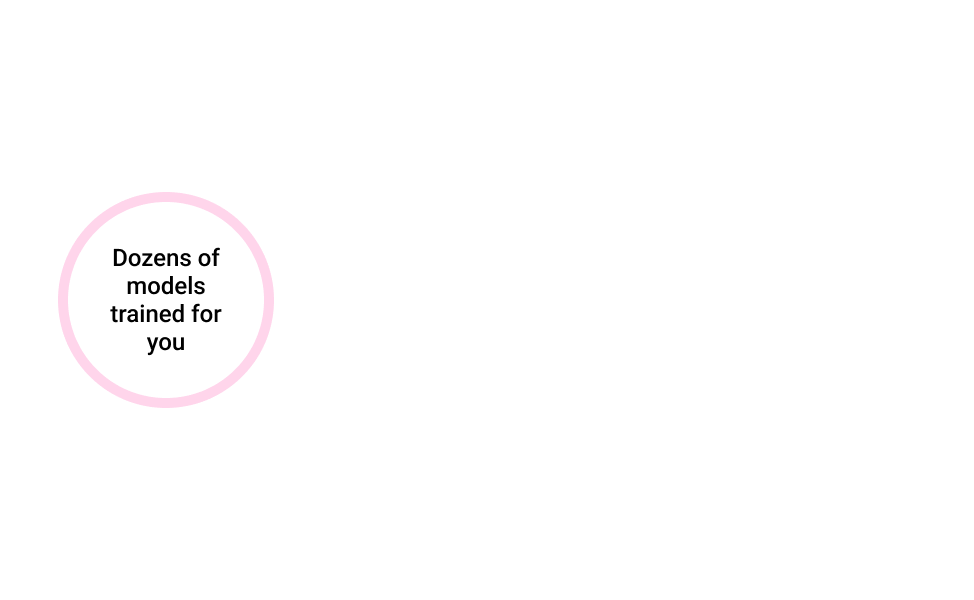
Automatically train and tune robust models via the world’s most advanced AutoML.
Automated pipeline does all ML for you: data preprocessing, foundation model fine-tuning, hyperparameter tuning, and model selection
ML models are used to diagnose data issues, and then can be re-trained on your corrected dataset with one click
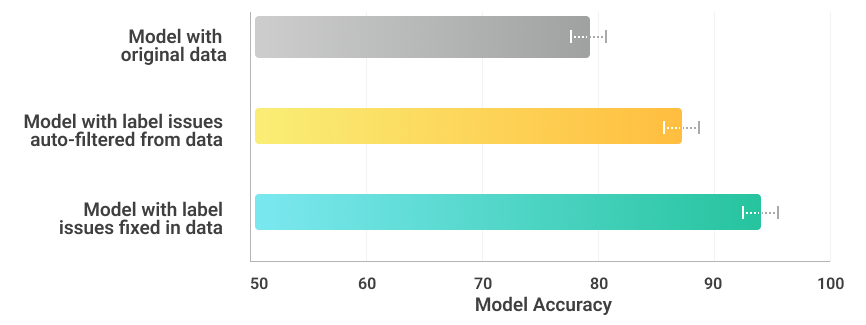
Seamless model deployment: Just a few clicks to get accurate predictions.
Train and deploy a robust ML model without writing code
Access real-time and batch inference through a web interface or REST API
Get predictions for unlabeled data or evaluate models on labeled test data
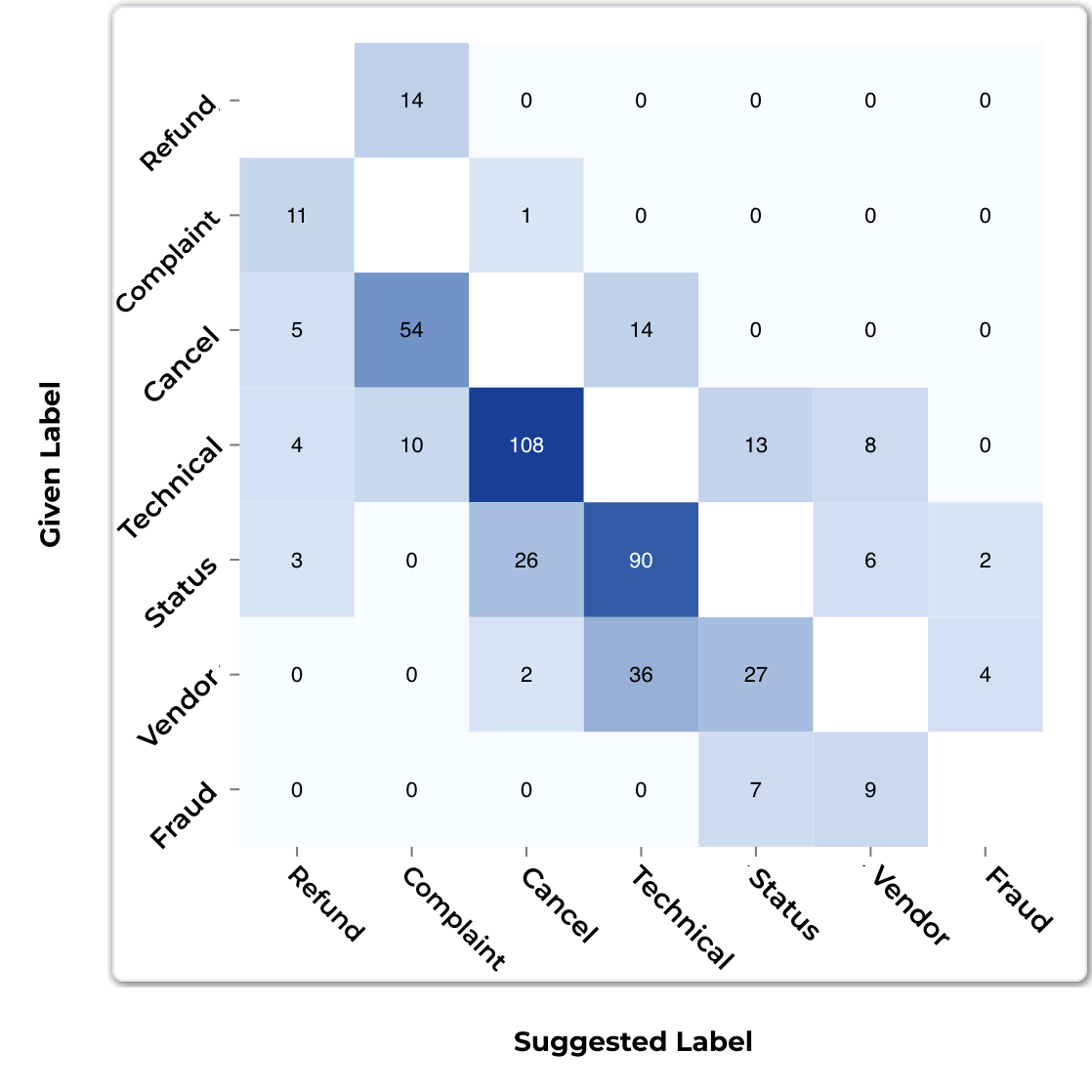
Explore analytics, summaries, and specific issues within your datasets.
Find the classes in your dataset with the most label issues
Explore the entire heatmap of suggested corrections for all classes in your dataset
Cleanlab Studio provides all of this information and more for free as soon as you upload your dataset
Testimonials from top organizations
using Cleanlab technology
Google used Cleanlab to find and fix label errors in millions of speech samples across different languages, to quantify annotator accuracy, and provide clean data for training speech models.
“Cleanlab is well-designed, scalable and theoretically grounded: it accurately finds data errors, even on well-known and established datasets. After using it for a successful pilot project at Google, Cleanlab is now one of my go-to libraries for dataset cleanup.”
One of the largest financial institutions in the world, Banco Bilbao Vizcaya Argentaria, uses Cleanlab to reduce label costs by over 98% and boost model accuracy by 28%.
“Cleanlab helped us reduce the uncertainty of noise in the tags. This process enabled us to train the model, update the training set, and optimize its performance. The goal was to reduce the number of labeled transactions and make the model more efficient, requiring less time and dedication. With the current model, we were able to improve accuracy by 28%, while reducing the number of labeled transactions required to train the model by more than 98%.”
Berkeley Research Group increases ML model accuracy by 15% and reduces time spent by 1/3 using Cleanlab Studio.
“We've started relying on Cleanlab to improve our ML and AI models at Berkeley Research Group LLC for over a month... I have to say, I'm impressed. Here's what we found: Increased model accuracy by 15%, Improved explainability & addressed performance impediments, Cut out training iterations by one-third, Overall performance improvement for our Data Science team.”
The Stakeholder Company reduced time spent by 8x in their ML data workflow by using Cleanlab to order data by label quality.
“We used Cleanlab to quickly validate one of our classifier models’ predictions for a dataset. This is typically a very time-consuming task since we would have to check thousands of examples by hand. However, since Cleanlab helped us identify the data points that were most likely to have label errors, we only had to inspect an eighth of our dataset to see that our model was problematic. We later realized that this was due to a post-processing error in the dataset — something that would otherwise have taken a much longer time to notice.”
Amazon AWS Principal Solutions Architect Cher Simon & Chief Evangelist Jeff Barr publish textbook that features Cleanlab in hands on exercises.
“Manually inspecting and fixing potential label errors can be time-consuming. We can train a better model using Cleanlab to filter noisy data.”
Learn more about how Amazon uses Cleanlab to improve the Alexa customer experiencehere
Why Cleanlab
built on the world’s cutting edge AutoML & AI layer
Prior to Cleanlab, Chief Scientist Jonas Mueller developed Amazon's AutoML platform, used today to train and deploy many models on AWS SageMaker
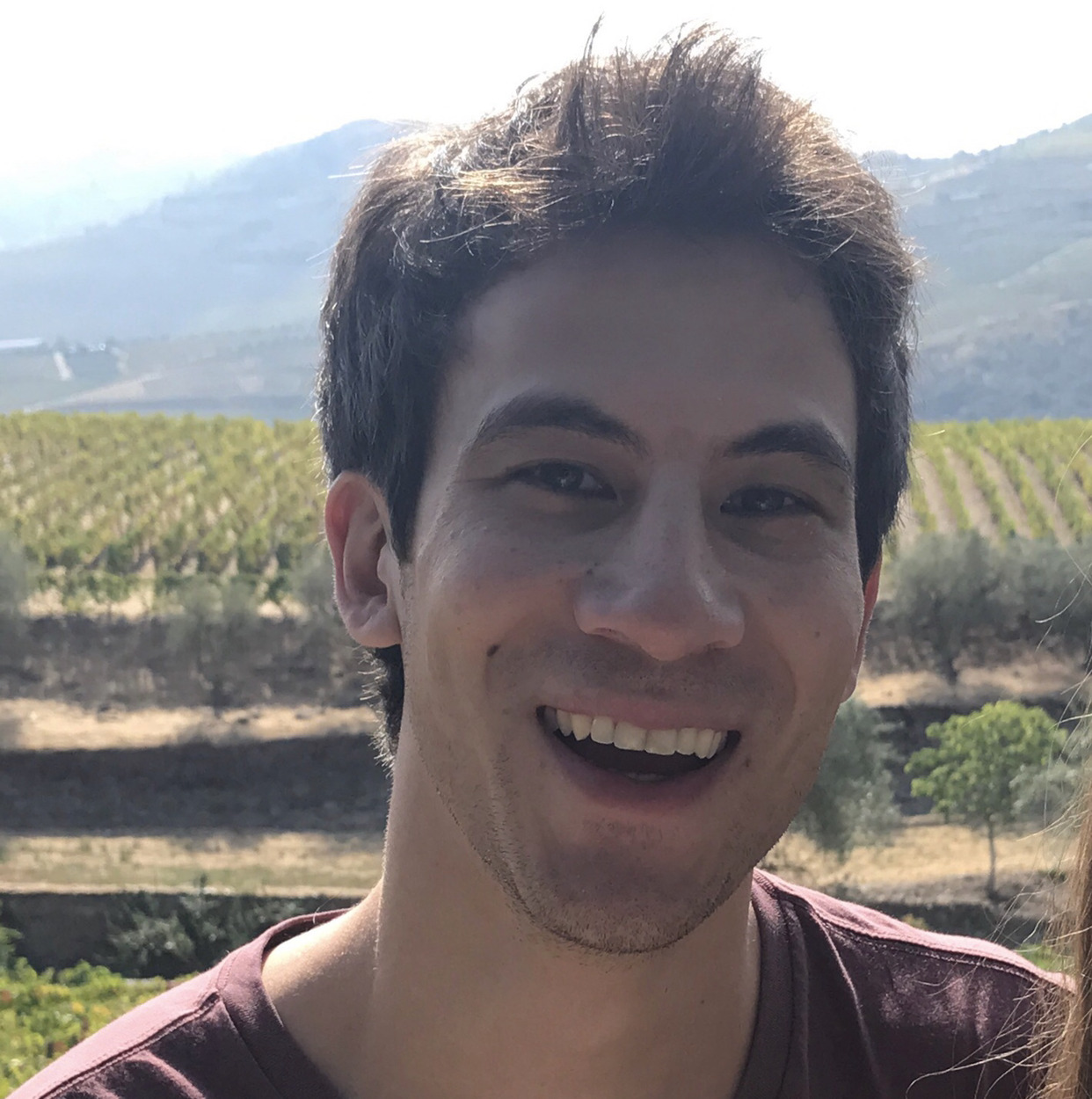
designed for security and scalability for enterprise from the ground up
Cleanlab’s Chief Technology Officer, Anish Athalye, is well cited for his PhD work at MIT in the world’s top systems lab (PDOS)
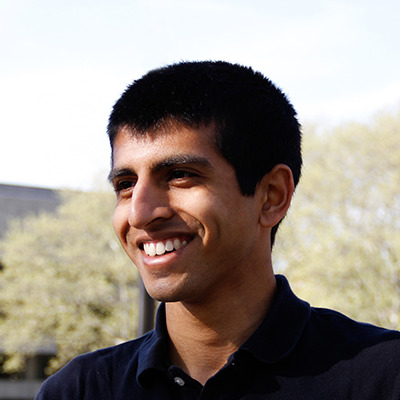
pioneered at MIT
Cleanlab’s Chief Executive Officer, Curtis Northcutt, invented confident learning during his PhD at MIT while working with the inventor of the quantum computer
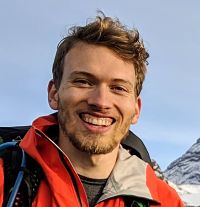
Enterprise Ready Data Ingestion
Cleanlab Studio interfaces with your ML pipelines, no matter where your data is stored.